Augmented Intelligence, machine learning, and analytics are increasingly deployed in service management systems and tool sets to enhance their performance. How and when they are used separately and in combination defines and/or limits how effective the AIOps application will be in improving service assurance processes from fault to customer experience management.
- Next generation, wide scope AIOps applications should employ:
Advanced anomaly detection to enable adoption to changes in seasonality, magnitude, and deviation over short intraday time periods - Stochastic models to reduce noise and detect performance issues and faults early
- Affinity analysis beyond simple temporal correlation to identify related events and define the root cause
- Probable cause determination using severity, entropy, and eccentricity metrics for every dimension to distinguish between symptoms and root cause
- Ontology reasoning to optimize performance management
ADVANCED ANOMALY DETECTION
Simple threshold-based anomaly detection simply does not work well in modern data centers or complex networks due to rapidly changing workloads and volumes, regardless of whether the thresholds are user-set or statistically learned. They are likely to trigger false positives during peak usage and heavy loads and miss true positives during quieter periods. Instead, more adaptive anomaly detection is required, one that continuously learns seasonality in load and usage, and triggers alerts based on deviation from expected behavior. Utilizing unsupervised machine learning, advanced anomaly detection relies on learning time-varying baselines on each metric and dimension as data is ingested, and continuously updating them as more data is collected. Triggering alerts based on deviations from learned baselines provides more robust alerting, i.e., capturing the significant anomalies occurring during low usages time periods, while reducing the false positive noise that often occurs during peak periods.
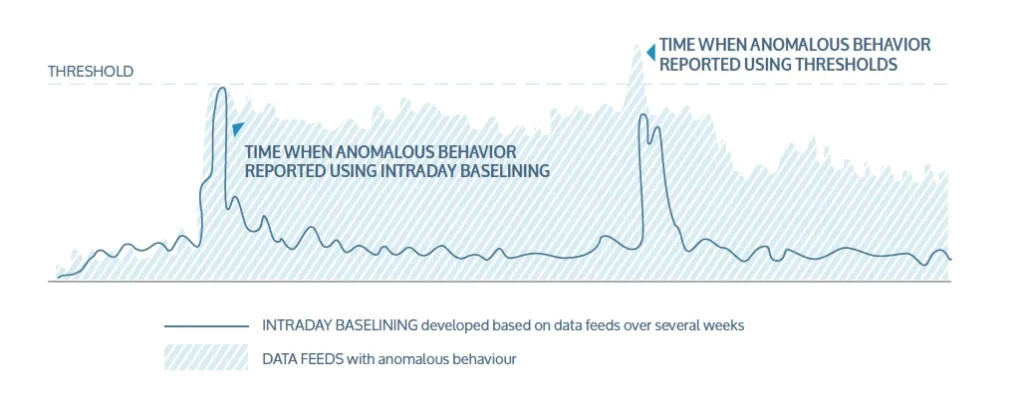
STOCHASTIC MODELS
Anomalous signals arising from the various event and metric streams being monitored are often transient, resulting from temporary usage spikes or statistical noise. These transient anomalies do not necessarily indicate a persistent problem. The ability to identify anomalies that are both significant and non-transient enables operations teams to focus on those problems that truly need fixes, and hence improves operational efficiency.
Stochastic models excel at separating signal from noise. For this reason, they are widely used on Wall Street to model the seemingly random fluctuations in market behavior and volatility, and predict when market conditions have changed. And for similar reasons, they are useful in the noisy world of data centers and IT operations. These models can continuously monitor and evaluate the behavior of every metric, event and entity looking for non- transient anomalies and suspicious changes in state that indicate an “incident” is occurring and needs correcting. Stochastic models correctly detect the patterns that other techniques will typically misclassify, identify late, or miss altogether.
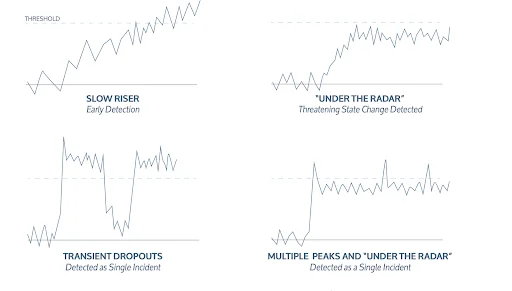
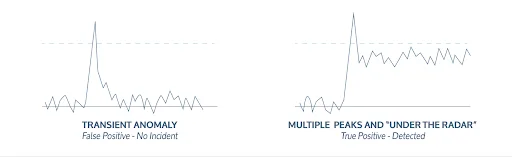
Stochastic models also allow for a dynamic “look-back” period to capture the point in time where the system first exhibited a detrimental change in behavior. This look-back period is likely to spot issues before the signal is declared to be an “incident” by most fault and performance management systems. Look back periods can even identify “slow risers” where the change in behavior takes a long time to manifest into a service-impacting Incident.
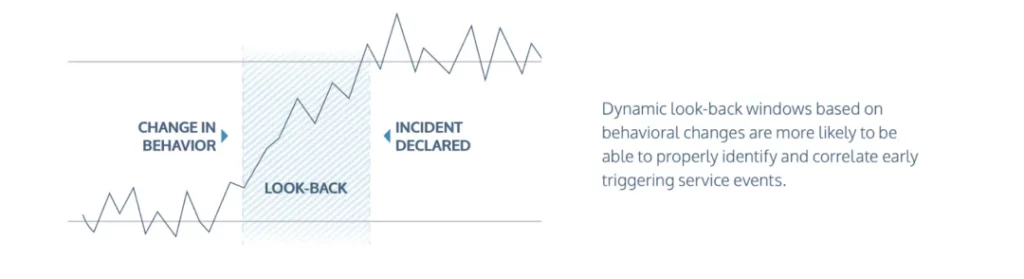
When considering a next generation AIOps application, data science is a clear differentiator.
How data science is used impacts:
- What processes and use cases can be optimized
- Their ability to uncover root cause and separate cause from symptoms across the service technology stack and across subsystems
- The speed at which performance issues and faults are detected
- The insight provided in order to accelerate not only response but resolution
Ask the difficult questions to understand the analytic strengths and weaknesses of AIOps.
To learn more click here.